The 11th IAPR International Conference on Biometrics
Tutorial#1: Biometric Spoofing and Anti-Spoofing: Presentation Attack Detection
Abstract
In biometrics, Presentation Attacks (PA also referred to as spoofing) are performed by falsifying the biometric trait and then presenting this falsified information to the biometric system, one such example is to fool a fingerprint system by copying the fingerprint of another person and creating an artificial or gummy finger which can then be presented to the biometric system to falsely gain access.
This is an issue that needs to be addressed urgently because it has recently been shown that conventional biometric techniques, such as fingerprints, face or voice, are vulnerable to presentation attacks. Hence, presentation attacks can be considered as one of the major obstacles preventing the adoption of biometric recognition in practical applications.
One of the main challenges in Presentation Attack Detection (PAD also referred to as antispoofing) is to find a set of features and models (mostly classifiers) that allows systems to effectively distinguish signals that were directly emitted by a human from those reproduced by an attacker. Generalization ability of PAD systems has been assessed for few biometrics (face or voice) showing the degradation in performance when specific features/models optimized using one database are used unchanged on another database. Cross-database experiments demonstrated the inability of current techniques to deal with unforeseen conditions.
For all these reasons, more research in biometric spoofing and anti-spoofing is needed and we hope that this tutorial will help researchers to bring forces in this exciting research area.
Program:
This tutorial will present:
- an introduction to the problem of presentation attacks,
- the vulnerability of some biometrics to presentation attacks,
- a review of some methods for presentation attack detection methods,
- a methodology for the evaluation of biometrics under presentation attacks,
- metrics for the evaluation of presentation attack detection,
- lessons learned from previous or ongoing competitions and projects.
We plan to propose before the tutorial, optional material to reproduce with no effort (no programming) experiments in face and voice PAD using the BEAT platform.
Instructor:
Sébastien Marcel received the Ph.D. degree in signal processing from Université de Rennes I in France (2000) at CNET, the research center of France Telecom (now Orange Labs). He is currently interested in pattern recognition and machine learning with a focus on biometrics security. He is a senior researcher at the Idiap Research Institute (CH), where he heads a research team and conducts research on face recognition, speaker recognition, vein recognition and presentation attack detection (anti-spoofing). In 2010, he was appointed Visiting Associate Professor at the University of Cagliari (IT) where he taught a series of lectures in face recognition. He is lecturer at the Ecole Polytechnique Fédérale de Lausanne (EPFL) where he is teaching on “Fundamentals in Statistical Pattern Recognition”. He serves on the Program Committee of several scientific journals and international conferences in pattern recognition and computer vision.
He is Associate Editor of IEEE Signal Processing Letters. He was Associate Editor of IEEE Transactions on Information Forensics and Security, a Co-editor of the “Handbook of Biometric Anti-Spoofing”, a Guest Editor of the IEEE Transactions on Information Forensics and Security Special Issue on “Biometric Spoofing and Countermeasures”, and Co-editor of the IEEE Signal Processing Magazine Special Issue on “Biometric Security and Privacy”. Finally he was the principal investigator of international research projects including MOBIO (EU FP7 Mobile Biometry), TABULA RASA (EU FP7 Trusted Biometrics under Spoofing Attacks) and BEAT (EU FP7 Biometrics Evaluation and Testing).
Webpage: http://www.idiap.ch/~marcel/professional/Publications.html#topics:Spoofing
Email: marcel@idiap.ch
Tutorial#2: Human-Device Interaction for Cognitive Behavioral Biometrics: Trends, Scenarios and Applications
Abstract:
Human interaction with electronic devices (laptops, tablets, mobile devices) by means of existing sensors (audio, video, keypad, touchpad, touch screen…) permits statistical modelling of gesture-driven activities, opening new fields and trends in behavioral biometrics. The use of keystroked text, handwritten information, finger trajectories on touchscreens or mouse dynamics allows continuous identification of users, not only for security purposes but also for user convenience in new application scenarios like e-health, websurfing, gaming, retail buying or mobile banking.
Program:
List of topics to be presented:
- Variability in human behavior and how to cope with it
- Cognitive behavioral biometrics: limits and capabilities
- Audio and speech biometrics
- Human Screen interaction: swiping and handwriting
- Keystroking and keypadding as input and device interaction
- Future trends and applications: some examples
Instructor:
Javier Ortega-Garcia got his Masters degree in Electrical Engineering in 1990, and his PhD degree in Electrical Engineering in 1996, both from Technical University of Madrid. He is a Full Professor at the Signal Processing Chair in Autonoma University of Madrid, where he leads the Biometrics and Data Pattern Analytics Lab (BiDA-Lab). His current research topics are focused in behavioral biometrics, specifically in pattern recognition techniques and signal processing for human-gesture modelling in security applications. He has published over 350 scientific and technical contributions (including book chapters, and journal and conference papers) in these fields. In 2004 he also was a founder of Agnitio, a spin-off company focused on speaker verification. He has been appointed as Chair in many related conferences, as the ISCA Odyssey International Conference on Speaker and Language Recognition 2004, the IAPR International Conference on Biometrics 2013 and the IEEE 51st International Carnahan Conference on Security Technology 2017, among others.
Web page: https://atvs.ii.uam.es/atvs/index
Email: javier.ortega@uam.es
Tutorial#3: Fingerprint Presentation Attacks Detection: Lessons Learned and a RoadMap to the Future (Slides)
Abstract:
More than 15 years have passed when, between 2001 and 2002, the first scholars pointed out the vulnerability of the fingerprint electronic capture devices to possible “attacks” by artificial replicas of the fingers, named “fake fingers” or “gummy fingers”, impossible to distinguish from “live fingers” without appropriate “detectors” or simply by visual inspection of the fingerprint image. From then, many possible countermeasures reported in journals, conference proceedings and international projects, have led to a more rigorous approach from the scientific community. This involved the initial terminology too: today, the terms “fingerprint vitality” or “fingerprint liveness” detection are included in the large field of the “presentation attacks” detection. The goal of this half-day tutorial is to explore the state of the art on this topic, emphasized by the long-time experience of the Biometric Unit of the PRA Lab (Pattern Recognition and Applications Laboratory) in this activity since from 2003. Our aim is to list and explain the main lessons learned, and trace a possible roadmap for future research works that we want to share with the tutorial participants.
Program:
- Problem statement: description and terminology
- Early terminology
- ISO/Standard terminology
- Hardware- and software-based approaches
- Fingerprint Presentation Attacks Detection taxonomy of approaches
- “Live-“ and “Fake-“ based methods
- Dynamic and static methods
- Textural methods
- Multi-modal methods
- Performance evaluation:
- International Fingerprint Liveness Detection Competition 2009-2017
- Other data set
- Lessons-learned from the PRA Lab experience
- Acquisitions of live and fake fingerprints: materials and methods
- Interoperability of FPAD
- User-specific FPAD
- Integration with verification systems
- Multi-modal PAD – meta-analysis
- A possible “roadmap”: open issues and the future of FPAD (including discussions with participants)
Instructor:
Gian Luca Marcialis is Assistant Professor of Computer Engineering at the University of Cagliari, Italy. He is currently habilitated as Associate Professor of Computer Engineering according to the Italian Ministry of Education, University and Research (MIUR). He obtained the MS degree in Electronic Engineering in 2000, and the Ph.D. degree in Computer Engineering in 2004. Since 2000 he joined the Pattern Recognition and Applications Laboratory (PRA Lab) of the Dept. of Electrical and Electronic Engineering, University of Cagliari, Italy, where he is currently director of the Lab’s Biometric Unit. His research interests are in the area of pattern recognition and its application to new and challenging tasks. During his career Gian Luca Marcialis has published more than one hundred papers on international journals, conferences and books (H-index is 28 according to Google Scholar). His main contributions are in the field of biometrics and in particular on the multi-modal fusion of classifiers for fingerprint classification and verification, the vulnerability assessment of multiple biometric systems, face recognition, adaptive biometric systems and fingerprint liveness detection, forensics, EEG signal processing. Gian Luca Marcialis acts as a reviewer for the vast majority of the international journals on pattern recognition and applications, as well as member of the programme committee of many IEEE and IAPR international conferences. He is involved in international and national research projects as responsible and team leader, and is also co-organizer of the International Competition of Fingerprint Liveness Detection (LivDet, http://livdet.diee.unica.it). He teaches B.Sc. and Ph.D. courses at University of Cagliari on the topic “Biometric technologies for information security”. Gian Luca Marcialis is a member of the IAPR (International Association for Pattern Recognition) and IEEE (International Association of Electrical and Electronic Engineers).
Web page: https://pralab.diee.unica.it/en/GianLucaMarcialis
E-mail: marcialis@diee.unica.it
Tutorial#4: Face Recognition in Deep Learning Era: Recent Progress and Some Trends (Slides Part 1, Part 2)
Abstract:
Thanks to the boom of deep learning, in the past 5 years, face recognition has undergone a big jump in terms of both technologies and applications. In this tutorial, Prof. Shan will firstly introduce the basic knowledge of Deep Convolutional Neural Network (DCNN), followed by its influence on all the component technologies of face recognition, including face detection, facial landmarks locating, face verification, and face identification. Then, he will take some efforts to introduce the blooming applications of face recognition in China, such as large-scale cross-matching of ID face photos for duplicate ID removal, face verification matching the inside-ID-card photo and the face of the card holder; and face verification matching an ID photo contaminated by rippling-curve and the face of the card holder. In the second part of this tutorial, Dr. Wiliem will introduce Generative Adversarial Network (GAN) and its variants, following by its application to face recognition, where GAN can be used in two ways: generating synthetic data, or transforming the query face into the domain where the face recognition model has been trained. Finally, some promising future research topics will be discussed.
Program:
- Basic knowledge of DCNN and how it influenced face recognition area;
- Typical successful face recognition applications in China and the techniques behind,
- Large-scale cross-matching of ID face photos to remove duplicate ID;
- 1:1 face verification: matching the inside-ID-card photo and the face of the card holder;
- 1:1 face verification: matching an ID photo contaminated by rippling-curve and the face of the card holder;
- GAN and its application to face recognition
- Brief introduction on GAN;
- Recent important works in GAN
- GAN for image-to-image translation
- GAN applications for face recognition
- Some promising future research topic
Instructor:
Shiguang Shan received Ph.D. degree in computer science from the Institute of Computing Technology (ICT), Chinese Academy of Sciences (CAS), Beijing, China, in 2004. He has been a full Professor of this institute since 2010 and now the deputy director of CAS Key Lab of Intelligent Information Processing. His research interests cover computer vision, pattern recognition, and machine learning. He has published more than 200 papers, with totally more than 12,000 citations (Google scholar). He served as Area Chairs for many international conferences including ICCV’11, ICPR’12, ACCV’12, FG’13, ICPR’14, ICASSP’14, ACCV’16, and FG’18. And he is Associate Editors of several journals including IEEE T-IP, Neurocomputing, CVIU, and PRL.
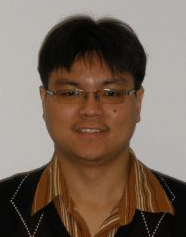